Given the significant proportion of the global population living in rural areas and the urgent need to address the depletion of natural resources such as water, air, and soil in these regions, which also contributes to climate change and diseases such as the Coronavirus pandemic [1], it is crucial to prioritise rural development alongside urban development, as emphasised by the Sustainable Development Goals (SDGs) and the guiding principle of the 2030 Agenda of ensuring that everyone is included [2]. The importance of rural development to the SDGs is underscored by the fact that over 3.4 billion people, or about 43% of the world's population, reside in rural areas, with a significant proportion residing in Asia and Africa. Nevertheless, rural populations differ widely from country to country; in some, such as the United States and Canada, most people reside in cities, whereas in others, like India and China, many people live in rural areas [2].
Based on the urban-rural typology of the European Union (EU) countries, nearly 45% of the EU's area in 2021 comprises predominantly rural regions. There have been relatively rapid population increases of at least 0.3% per year in 108 predominantly rural EU regions since 2015. In contrast, the population of 155 predominantly rural regions fell relatively fast, less than −0.3% per year [3]. Observing the Western Balkan countries, as of 2020, the population living in rural areas is approximately 40.4% [4]. Currently, 44% of Serbia's population lives in rural areas [4]. Statistics on rural populations and the varying rates of population growth in different regions worldwide highlight the need for a tailored approach to rural development. Furthermore, within regions characterised by limited water availability, researching the ecological consequences linked to water utilisation in urban, industrial, and agricultural sectors and the depletion of local freshwater resources may contribute to a significant detriment in sustainability [5]. In addition to addressing public health challenges and mitigating climate change and resource depletion, this is part of a larger initiative to achieve the SDGs, notably SDG 6 − universal access to safe drinking water and sanitation [6].
Water resources play a critical role in public water supply systems due to their fundamental importance in sustaining human life and supporting various societal and economic activities [7]. It is known that water availability and quality are threatened by climate change, pollution, and overuse [8]. Water is essential for maintaining proper hygiene and sanitation, as access to clean and safe drinking water is directly linked to public health outcomes, promoting overall well-being as repeatedly emphasised in the EU Water framework directive [9]. Public water supply management involves environmental sustainability considerations, including minimising water pollution from nutrients, preserving aquatic habitats, and safeguarding natural water sources [10]. Water resources are fundamental to the health of both aquatic and terrestrial ecosystems, serving as a cornerstone for a diverse range of biological and ecological processes. Therefore, maintaining a sustainable balance, especially in surface water resources, is imperative for preserving aquatic ecosystems, biodiversity, and overall ecological integrity [11].
Equally important is the role of water resources management in climate change [12], as altered weather patterns, droughts, and extreme weather phenomena increasingly challenge the availability and quality of potable water [13]. For instance, the severe drought in California from 2012 to 2016 significantly strained water supplies [8]. Moreover, regions dependent on seasonal snowpack for water are at risk as rising temperatures contribute to diminished snowpack levels, affecting the quantity and quality of water supplies [14]. Consequently, fluctuations in river flow volumes and the ensuing variations in raw water quality present substantial challenges to public water supply systems in light of climate-induced changes [15].
The need for a customised approach in rural development is underscored by the challenges associated with securing access to clean and safe drinking water [16]. The importance of this issue transcends mere biological necessities, embracing a broad spectrum of socio-economic impacts that directly affect SDGs, notably SDG 6, in rural areas [17]. Such areas are particularly susceptible to water quality degradation, a vulnerability exacerbated in areas prone to extreme hydrological events [18]. This issue is paramount in adapting small-scale public water supply systems prevalent in rural settings, which serve populations ranging from a few thousand to tens of thousands. These systems often rely on surface water as the primary source, necessitating subsequent treatment to meet consumption standards [11]. Rural water resources are used for various agricultural and economic objectives, including irrigation, aquaculture, landscape development, and aquatic species preservation in tropical and temperate countries. These uses depend on the different physical properties of water bodies, their water supply, and the availability of water [19]. With this understanding, monitoring water quality parameters during extreme events becomes even more pronounced as it plays a vital role in maintaining ecological equilibrium and fostering sustainable economic development [20]. Each adaptation endeavour necessitates specific adjustments encompassing technical, organisational, and individual dimensions, accompanied by consequential restructuring and transformation procedures. Typically, these adaptations demand substantial material resources and suitable cognitive capabilities, frequently limited in small-scale public water supply systems. Consequently, it becomes imperative to characterise, quantify, and partially forecast variations in chosen raw water quality parameters in response to alterations in volumetric flows over a defined timeframe. Observing discernible patterns of these changes is significant for optimising the raw water treatment process [21].
Emerging research has provided compelling evidence regarding the substantial influence of extreme hydrological events on the water quality parameters of untreated water sources [22]. These events can cause surface water runoff that may carry pollutants and sediments into drinking water sources. Numerous fields, including climatology, earth science, hydrology, ecology, engineering, and social science, have conducted substantial research on extreme occurrences [23]. Establishing threshold values for flow extent, which identify high and low flows, is necessary to define extreme hydrological events. When these predetermined thresholds are exceeded, it signifies an exceptional hydrological event. Floods resulting from continuous precipitation surpass typical multi-year flow rates, while floods triggered by heavy storm precipitation lead to elevated water levels. Continuous precipitation noticeably impacts both water level fluctuations and water quality deterioration. Furthermore, the analysis of dry spells primarily addresses the issue of low water levels [23].
Research highlights that extreme hydrological events can significantly impact water quality in rural and urban catchments, altering concentrations of pollutants and nutrients due to changes in water flow dynamics. Horne [24] analysed the management of extreme hydrological events in the Australian urban water systems context, focusing on the issues that smaller service providers confront while meeting community expectations during such occurrences. It emphasises the significance of governance reforms and appropriate management measures to balance water flow and quality during harsh conditions. Xiong and Wang [25] developed a cross-scale approach linking water-flow-regulating ecosystem services at a macro scale with the assessment of the constructed urban environment at a micro scale. This study, conducted in Bochum, Germany, revealed the urban-rural difference in water-flow-regulating services and suggested ways to bridge the science-practice gap in water management. McGrane et al. [26] studied the hydrological and water quality response to extreme storm events in a UK catchment with a distinct rural-urban gradient. They found that rural areas exhibited slower hydrological responses and different water quality dynamics than urban areas, indicating that land use significantly influences the interdependence between water flow and quality during extreme events. Li et al. [27] assessed the hydrological response to an extreme meteorological drought event in the Baiyangdian basin, using base flow isolation methods to analyse streamflow and base flow. The study found a time lag between the end of meteorological and hydrological drought events, with baseflow recovery lagging behind streamflow recovery. De Bastos et al. [28] conducted a study in Southern Brazil to identify pollution sources in a rural headwater catchment. They emphasised the importance of multi-scale water quality monitoring and the impact of agricultural activity on water quality during extreme hydrological events. Wang et al. [29] evaluated the individual and combined impacts of land use and climate change on extreme hydrological events in the Xitiaoxi River Basin, China. Their findings suggest that the region will experience more severe extreme hydrological events due to these changes. Puczko and Jekatierynczuk-Rudczyk [23] presented a study on the influence of hydrometeorological extreme events and urban catchment on water quality in small rivers in Northeast Poland, highlighting the significant impact of these events on water quality parameters.
South-eastern Serbia, for instance, is a region where extreme hydrological events occur frequently, affecting the quality and availability of drinking water. The impact of such extreme hydrological events on drinking water quality in rural areas like Vlasotince is an issue that requires close attention due to its vulnerability. Firstly, the literature lacks studies that examine the interdependence between water flow and multiple water quality parameters during extreme hydrological events. Secondly, there is a need to explore the extent of the impact of such events on rural water supply systems, which are often under-resourced and less resilient to such shocks. Consequently, it is critical to develop appropriate strategies that mitigate these risks through rigorous research-based solutions to ensure safe access to clean drinking water in affected regions. In this context, Figure 1 presents a conceptual model for understanding the interactions between various natural and anthropogenic factors and their effects on water quality in a given spatial and temporal context. The central theme of the diagram is "Qualitative and quantitative oscillations ", suggesting the focus on both the quality and quantity variations in water bodies. This conceptual framework provides a basis for examining the complex dynamics influencing water quality, thus informing the development of effective strategies for safeguarding drinking water resources in rural areas prone to extreme hydrological events.
A conceptual framework for assessing the oscillations in water quality due to natural and anthropogenic influences
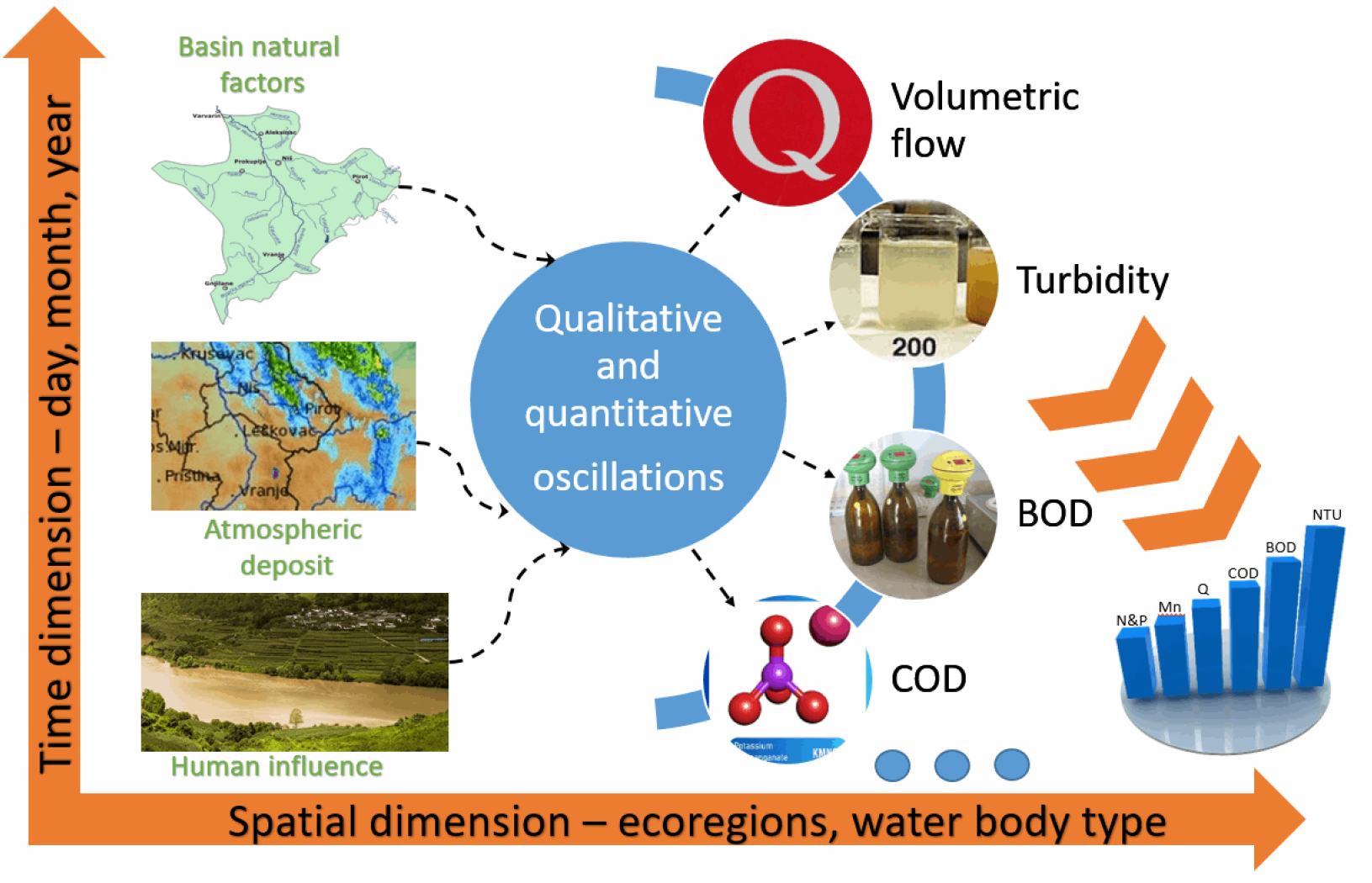
The orange vertical arrow, labelled "Time dimension – day, month, year ", indicates that water bodies' qualitative and quantitative characteristics are measured over various time scales, ranging from daily to annual assessments. The horizontal arrow, labelled "Spatial dimension – ecoregions, water body type ", refers to the geographical location of the water body, such as ecoregions or specific water body types (e.g., rivers, lakes, estuaries). The diagram identifies three primary factors influencing these oscillations: "Basin natural factors ", which are illustrated by a map, suggesting that the natural features of a drainage basin (topographical, geological, and others) affect water characteristics; "Atmospheric deposit ", represented by a satellite image, possibly indicating the impact of atmospheric conditions or deposits (like precipitation or pollutants) on water quality; "Human influence ", shown by a photograph of a landscape with human activity (agriculture), indicating the anthropogenic effects on water bodies. There are also some water quality parameters such as turbidity – represented by a visual of water clarity or cloudiness; chemical oxygen demand (COD) – indicated by a molecular structure and a graph, referencing the amount of oxygen required to chemically oxidise organic and inorganic pollutants; biological oxygen demand (BOD) – illustrated with a graph and laboratory bottles, suggesting the measurement of the amount of oxygen required by bacteria to decompose organic matter in water; Volumetric flow which is symbolised by a flask with a measurement marking, referring to the amount of water flowing through a particular point over time. The bar graph at the bottom right corner details these indicators (with additional parameters like nitrogen and phosphorus concentrations and possibly manganese), correlating them with the volumetric flow.
This study aims to investigate the qualitative and quantitative fluctuations in raw water quality by analysing the interplay between natural basin characteristics and extreme hydrological events across various spatial dimensions, such as types of water bodies and temporal scales, within a five-year study period (2014–2018), focusing on a case study in Vlasotince, south-eastern Serbia. The research will serve as a model for understanding these dynamics in other rural settings with similar basin characteristics where data collection is challenging. It seeks to explore how these factors contribute to the variability in ten raw water quality parameters, including nitrates (NO3-), turbidity, COD, and colour. Additionally, the study will examine the oscillations in water flow within the Vlasina River basin, the principal raw water source for the Vlasotince water supply system. This comprehensive evaluation aims to develop frameworks for sustainable water resource management and policy formulation by identifying pivotal factors influencing water quality and delineating the interrelations between extreme hydrological events and raw water quality, offering insights that can improve the management and resilience of rural water supply systems globally. Ultimately, this research endeavours to augment the current understanding of the impacts of extreme hydrological events on drinking water quality in rural settings, thereby addressing existing literature gaps and offering insights into the management and resilience of rural water supply systems.
To ascertain the variations in water quality parameters based on the water flow rate in periods of extreme hydrological events in Vlasotince, an analysis of variance (ANOVA) and post hoc Tukey's HSD (Honestly Significant Difference) test was used. Post hoc tests are crucial in the ANOVA context [30]. ANOVA is employed to examine the equality of means across three or more groups; the emergence of statistically significant outcomes suggests that not all group means are equivalent [31]. Nevertheless, ANOVA alone does not discern the specific pairwise differences among means deemed significant. This research also employs post hoc tests to explore and identify such differences while maintaining control over the experiment-wise error rate. Such tests allow for a comprehensive examination of distinctions among multiple groups, thereby enhancing the understanding of the overall experimental results.
ANOVA is a common technique [32] used in scientific research to compare means across multiple groups or treatments. By analysing all the many effects (also referred to as sources of variation) acting simultaneously on the response, this statistical technique determines which parameters are statistically significant and how much they contribute to the response variability [33]. For instance, such statistical analyses were commonly used to optimise the water quality monitoring network of water pollution caused by fish farms [34], to investigate the regional distributional pattern of groundwater quality characteristics [30], to quantify the extent of land use influence on river water quality [35], among others. In this case, the groups are the different levels of water flow rate, and the response variable is the water quality parameter. The water flow rate is the independent variable, and it is divided into three categories:
Minimum flow rates (Qmin) between 1.33 and 2.52,
Average flow rates (Qavg) between 2.53 and 10.10, and
Maximum flow rates (Qmax) between 10.11 and 133.00.
Each category represents a specific range of water flow rates. These categories are used to establish groups of the data for analysis and compare the water quality parameters across different flow rate intensities. The dependent variables, on the other hand, are the raw water quality parameters. However, ANOVA only indicates whether there are statistically significant differences between groups; it does not specify which groups differ from each other. Here, post hoc Tukey's HSD test comes in. A post hoc Tukey's HSD test was used to analyse and identify the specific levels of water flow intensity at which significant differences in water quality occur [23]. The post hoc Tukey's HSD test is a typical statistical procedure used in research to compare different groups and determine whether pairs of values differ significantly. It aims to provide insights into the specific thresholds of water flow intensity that result in notable disparities in the measured water quality parameters.
Hence, these techniques aid in determining which water flow rates exhibit statistically significant variances in water quality parameters and can be extended to evaluate water bodies categorised as good and moderate under the EU's Water Framework Directive (WFD) [36]. This methodology applies to water bodies sharing comparable geographical, hydrological, and climatic attributes, guaranteeing a thorough comprehension of their water quality trends [37]. Employing the previously described approach to these water bodies makes identifying statistically significant distinctions in water quality parameters possible, thereby supporting informed decisions in water resource management, environmental conservation initiatives, and decision-making processes concerning water quality enhancement.
This study used the Vlasina River basin in the Vlasotince region, south-eastern Serbia, as the case area. Vlasotince is located in south-eastern Serbia and covers the area of the lower and middle basin of the Vlasina River and the slopes of the Suva mountain, as shown in Figure 2 [38], [39].
The Vlasina River is classified as a moderate-sized watercourse and part of the Danube River watershed [40], [41]. With a surface area of 308 km², the municipality of Vlasotince is home to 25,849 inhabitants, according to the data from the Republic Statistical Office and the census conducted in 2022 [42]. The Vlasina River is the largest watercourse in the municipality of Vlasotince. It is a right tributary of the South Morava River, the second largest river in terms of size, with a length of 70 km. It originates from the Vlasina Plateau and flows through the Surdulica, Crna Trava, Vlasotince, and Leskovac regions. The most important tributaries in the Vlasotince area include the Tegošnica, Lužnica, Pusta River, Bistrica, and Rastavnica rivers [38]. About 165 km2 of Vlasotince's total land area, or about 53.6%, comprises agricultural land. The distribution, methods of use, and productivity levels of the agricultural land in this area show great variation [43].
The Vlasina River basin location: The Republic of Serbia in the map of Europe (a), Vlasotince area (b), Vlasina River basin [40] (c)
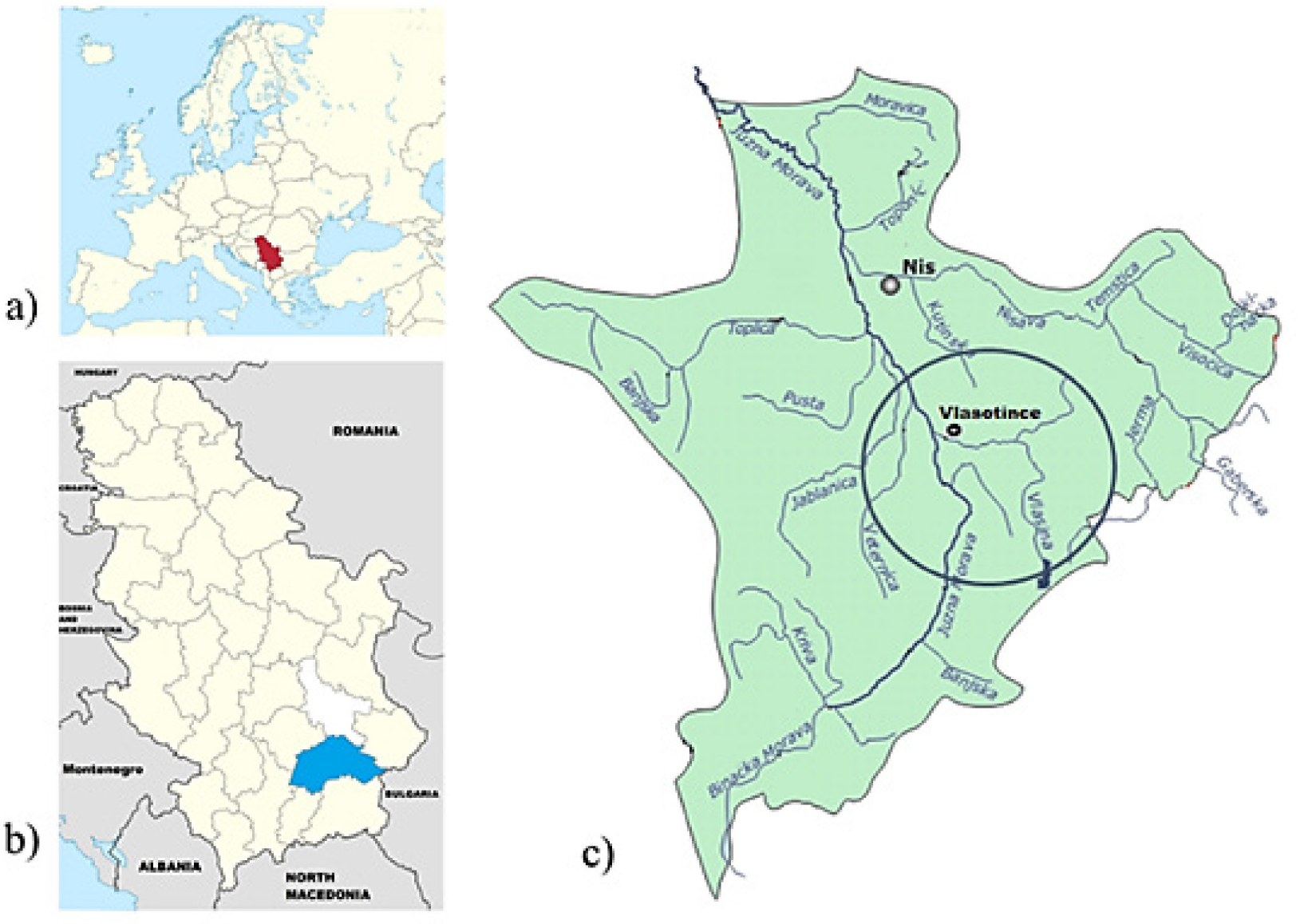
The fundamental characteristic of water quality in the Vlasina River basin is the absence of significant water pollution that could jeopardise its primary purpose of providing water supply to settlements. However, the most common deviations primarily involve organic matter, as the COD indicates [44]. Polluted raw water is exclusively associated with precipitation and upstream runoff, specifically the intensity and duration of rainfall, as well as the period of snowmelt. The variability in raw water quality occurs throughout the day, exhibiting significant fluctuations ranging from 3 to 5 times. The peak pollution event occurs approximately 2 to 3 hours after the onset of rainfall, indicating that contamination originates from the upstream catchment area within 5 to 15 km from the raw water intake. During extreme hydrological events, pollution levels surpass a COD of 100 mg/l or higher [44].
Furthermore, it is important to note that over the past 15 years, there has been a significant increase in forest logging activities within the Vlasina River watershed, resulting in intensified erosion of the surrounding land and elevated pollution levels from humic substances [44]. This occurrence is not exclusive to this particular location but rather a common phenomenon observed in many surface water supply areas across the Republic of Serbia. The extensive logging practices have led to detrimental environmental consequences, including soil erosion and increased sedimentation in water bodies, which can negatively affect water quality and aquatic ecosystems. It is crucial to address and mitigate the adverse effects of such practices by implementing sustainable forestry management strategies and promoting environmentally conscious approaches to ensure the long-term preservation of the watershed's ecological integrity.
The observed area encompasses the lower part of the Vlasina River basin. The entire basin is characterised by a highly fragmented terrain [38], with numerous tributaries and a dense hydrographic network. The basin takes on a fan-shaped pattern, with extremely steep slopes in the upper part, resulting in a rapid runoff concentration during heavy precipitation. The precipitation in this area is notably intense, particularly in the upper and middle reaches of the basin. These distinctive features collectively indicate the potential occurrence of sudden floods, short-duration waves, and significant destructive capacity [44]. The Vlasina River is the main watercourse in the area, characterised by flash floods. Its tributaries, such as the Pusta River and Lužnica, contribute to its size and potential for destructive force. Other smaller streams, including Stojkovačka Valley, Crnobarski Stream, and Pušina Valley, also pose a flash flood risk. The gentle slopes in this area result in sediment deposition from the upper parts of the basin into the Vlasina River and its tributaries [44].
A moderate-continental climate characterises the observed area. It is characterised by warm and dry summers, while winters are moderately cold with relatively low snowfall. The average annual air temperature is a fundamental indicator of the thermal regime, and it stands at 11.5 °C, following a consistent annual pattern with a clear minimum in January and a maximum in August [44]. The degree of air saturation with water vapour follows a normal annual trend, with maximum humidity in December−January and minimum values in August. The territory of Vlasotince receives varying amounts of precipitation throughout the year, ranging from 44 to 80.6 mm. The precipitation regime in the municipality is characterised by a peak in late spring and early summer (May and June), while the minimum precipitation occurs in late summer and early autumn (September and October). It can be concluded that this represents one variant of a continental precipitation regime [44].
The daily hydrological data were obtained from the database of the Republic Hydrometeorological Institute of Serbia, specifically from the surface water station in the Vlasina River basin located in Vlasotince. This station is part of the South Morava River basin, which covers an area of 879 km2. The water flow data were collected from 2014 to 2018, encompassing five years. This timeframe allowed for a thorough examination of the water flow patterns and fluctuations over a sufficiently long time interval. Collecting data over multiple years enabled capturing seasonal and annual fluctuations in water flow intensity, providing a more robust understanding of the hydrological dynamics in the study area. The dataset is valuable for investigating the relationships between water flow intensity and various water quality parameters, advancing knowledge in hydrological research and facilitating informed decision-making in water resource management.
The raw water quality data were acquired from the public water supply utility "Nerezine" in Vlasotince for the same period spanning from 2014 to 2018. These data were collected to assess the quality of the untreated water sourced from the utility. The water supply stations monitored and reported up to 11 water-related parameters, providing near real-time information daily. The parameters that were included in this study are ammonia (NH3), nitrates (NO3-), nitrites (NO2-), turbidity, COD, iron, manganese, pH value, colour, and aluminium. These parameters encompass water quality aspects that are crucial for evaluating the safety and suitability of the raw water for consumption and subsequent treatment processes during extreme hydrological phenomena.
Furthermore, to comprehensively investigate the interplay between water flow intensity and water quality parameters, it is essential to connect the hydrological data obtained from the Republic Hydrometeorological Institute of Serbia with the raw water quality data obtained from the public water supply utility "Nerezine" in Vlasotince. This integration allows for a comprehensive analysis of the relationships between water flow dynamics and water quality parameters. It enables a holistic understanding of the hydrological processes and their impact on raw water quality during extreme hydrological events.
As previously stated, the chosen statistical technique enables the comparison of means across several groups or levels of a single independent variable. It was feasible to assess whether there were significant differences in water quality parameters between different water flow rates using ANOVA. This type of analysis is critical for understanding how variations in flow rate affect water quality in a water delivery system [45].
The variables incorporated within the scope of this investigation comprise NH3, NO3-, NO2-, turbidity, COD, iron, manganese, pH levels, colour, and aluminium concentrations. This array of parameters encapsulates multifaceted dimensions of water quality. It assumes paramount significance in assessing the portability and appropriateness of untreated water for consumption, alongside its viability for subsequent treatment procedures, particularly in the face of extreme hydrological occurrences. These parameters collectively serve as critical indicators in discerning water resources' overall condition and safety standards, thereby underlining their pivotal role in ensuring public health and environmental sustainability amidst fluctuating hydrological dynamics.
Table 1 illustrates the dynamic variations in raw water quality parameters (e.g., turbidity, COD) attributed to the fluctuating intensity of water flow within the Vlasotince region. Table 1 defines N as the sample size. M denotes a specific group's mean or average value within the dataset. SD indicates the standard deviation, a statistical metric quantifying the extent of dispersion or variability within a set of values. The F-statistic (F), used in ANOVA, assesses significant differences between the means of two or more groups. The numbers in parentheses (2, 1820) represent the degrees of freedom associated with the F. The first value (2) indicates the number of compared groups. The second value (1820) represents the total degrees of freedom for error. Finally, the p-value (p) signifies the probability of random chance causing the observed results. Equipped with this knowledge of the statistical notations, Table 1 is a comprehensive reference for understanding the statistical nuances associated with the depicted fluctuations in raw water quality within the Vlasotince region. According to the intensity of the water flow, there is a significant difference in the turbidity of the water, F (2, 1820) = 89.72, p = 0.00.
Differences in water quality depending on the intensity of water flow in Vlasotince
Water quality parameter | Units | Water flow | N | M | SD | F | p |
---|---|---|---|---|---|---|---|
Turbidity | [NTU] | Minimum | 454 | 11.546 | 26.501 | 89.724 | 0.000 |
Average | 916 | 30.448 | 61.361 | ||||
Maximum | 453 | 87.138 | 156.145 | ||||
COD | [mg/l] | Minimum | 454 | 7.948 | 6.841 | 79.071 | 0.000 |
Average | 916 | 11.069 | 8.642 | ||||
Maximum | 453 | 16.234 | 14.504 | ||||
Ammonia | [mg/l] | Minimum | 411 | 0.035 | 0.040 | 5.706 | 0.003 |
Average | 789 | 0.051 | 0.106 | ||||
Maximum | 398 | 0.044 | 0.049 | ||||
Nitrates | [mg/l] | Minimum | 417 | 1.281 | 0.497 | 147.654 | 0.000 |
Average | 788 | 1.637 | 0.766 | ||||
Maximum | 402 | 2.126 | 0.768 | ||||
Nitrites | [mg/l] | Minimum | 417 | 0.002 | 0.002 | 50.845 | 0.000 |
Average | 791 | 0.004 | 0.004 | ||||
Maximum | 401 | 0.004 | 0.003 | ||||
Iron | [mg/l] | Minimum | 417 | 0.116 | 0.192 | 72.526 | 0.000 |
Average | 785 | 0.176 | 0.246 | ||||
Maximum | 386 | 0.345 | 0.407 | ||||
Manganese | [mg/l] | Minimum | 416 | 0.039 | 0.070 | 54.465 | 0.000 |
Average | 785 | 0.064 | 0.107 | ||||
Maximum | 394 | 0.132 | 0.209 | ||||
pH value | [-] | Minimum | 454 | 7.837 | 0.392 | 6.122 | 0.002 |
Average | 879 | 7.891 | 0.239 | ||||
Maximum | 432 | 7.894 | 0.263 | ||||
Colour | [PCU] | Minimum | 445 | 13.433 | 10.708 | 271.973 | 0.000 |
Average | 869 | 20.685 | 12.929 | ||||
Maximum | 379 | 35.231 | 17.539 | ||||
Aluminium | [mg/l] | Minimum | 416 | 0.145 | 0.069 | 26.596 | 0.000 |
Average | 784 | 0.166 | 0.091 | ||||
Maximum | 392 | 0.193 | 0.115 |
A post hoc Tukey HSD test was utilised to identify the water flow rates at which the discrepancies exist. In comparison to minimal water flow (M = 11.55 NTU, SD = 26.50) and average water flow (M = 30.45 NTU, SD = 61.36), water turbidity is higher at maximum water flow (M = 87.14 NTU, SD = 156.15); p < 0.05. When compared to the average intensity of water flow (M = 30.45 NTU, SD = 61.36), the water turbidity at the minimum water flow (M = 11.55 NTU, SD = 26.50) is lower; p < 0.05. Figure 3 presents fluctuations in water quality parameters concerning flow intensity, specifically focusing on turbidity in the depicted scenario.
There is a significant difference in the concentration of COD depending on the intensity of water flow, F (2, 1820) = 79.07, p = 0.00. The concentration of COD during maximum water flow (M = 16.23 mg/l, SD = 14.50) is higher compared to when there is minimum water flow (M = 7.95 mg/l, SD = 6.84) and when there is an average intensity of water flow (M = 11.07 mg/l, SD = 8.64); p < 0.05.
The average water turbidity concentration
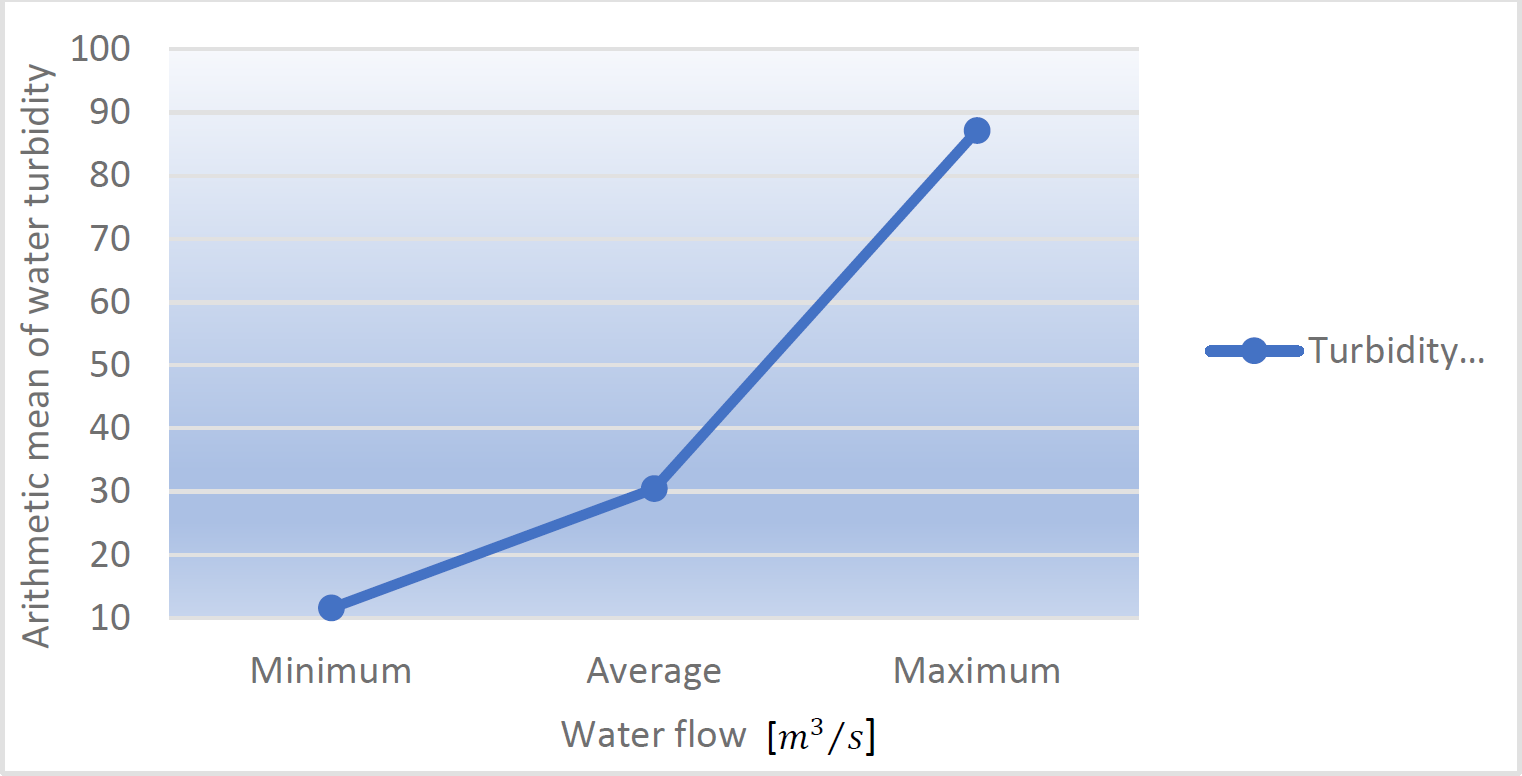
The concentration of COD during minimum water flow (M = 7.95 mg/l, SD = 6.84) is lower compared to the average intensity of water flow (M = 11.07 mg/l, SD = 8.64); p < 0.05. Figure 4 depicts alterations in parameters related to water quality as influenced by the intensity of water flow, specifically focusing on COD concentration in this instance.
The average COD concentration
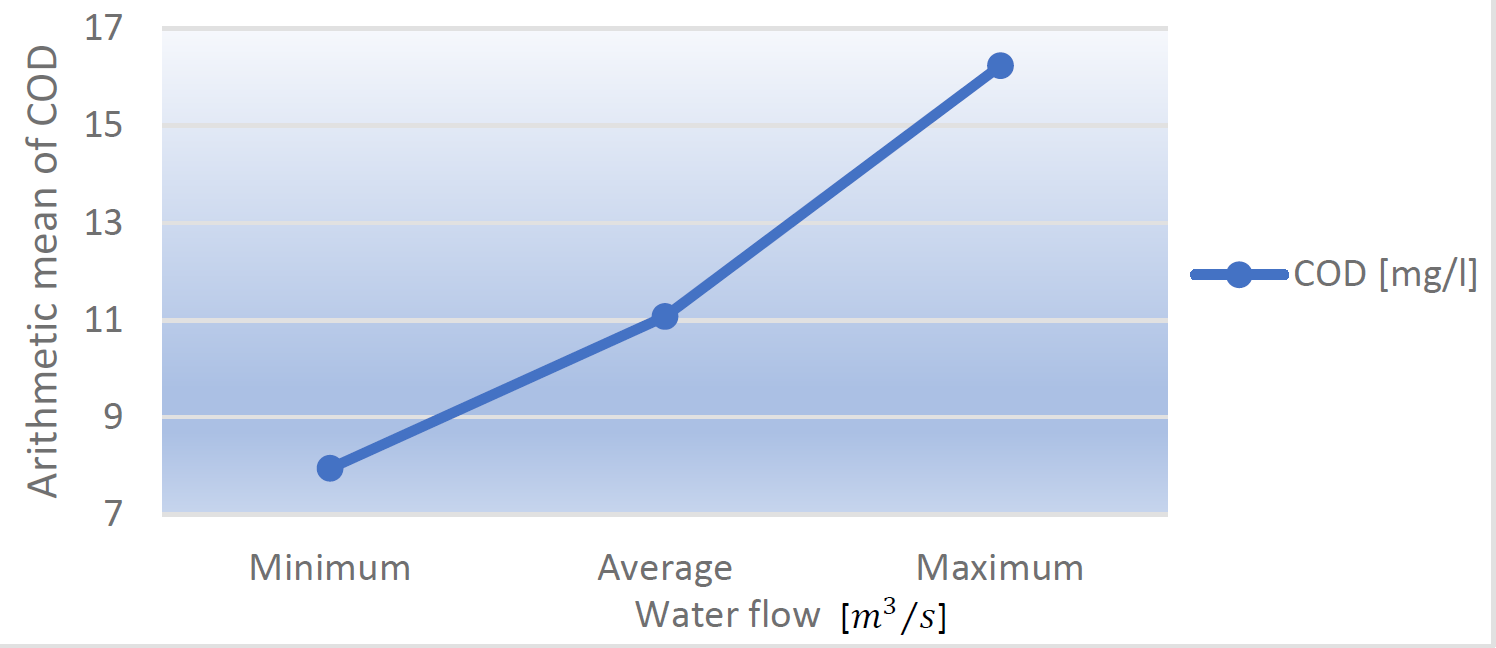
A difference in the ammonia concentration in water has been observed depending on the intensity of water flow, F (2, 1595) = 5.71, p = 0.00. The concentration of ammonia in water during minimum water flow (M = 0.04 mg/l, SD = 0.04) is lower compared to the average intensity of water flow (M = 0.05 mg/l, SD = 0.11); p < 0.05. The ammonia concentration in water during maximum water flow does not significantly differ from the average or minimum water flow; p > 0.05. There is a significant difference in the concentration of nitrates in water depending on the intensity of water flow, F (2, 1606) = 147.65, p = 0.00. The concentration of nitrates in water during maximum water flow (M = 2.13 mg/l, SD = 0.77) is higher compared to when there is minimum water flow (M = 1.28 mg/l, SD = 0.50) and when there is an average intensity of water flow (M = 1.64 mg/l, SD = 0.77); p < 0.05. The concentration of nitrates in water during minimum water flow (M = 1.28 mg/l, SD = 0.50) is lower compared to the average intensity of water flow (M = 1.64 mg/l, SD = 0.77); p < 0.05. Figure 5 illustrates the fluctuations in water quality attributes concerning flow intensity, specifically focusing on NO3- concentration in this context.
The average nitrates concentration
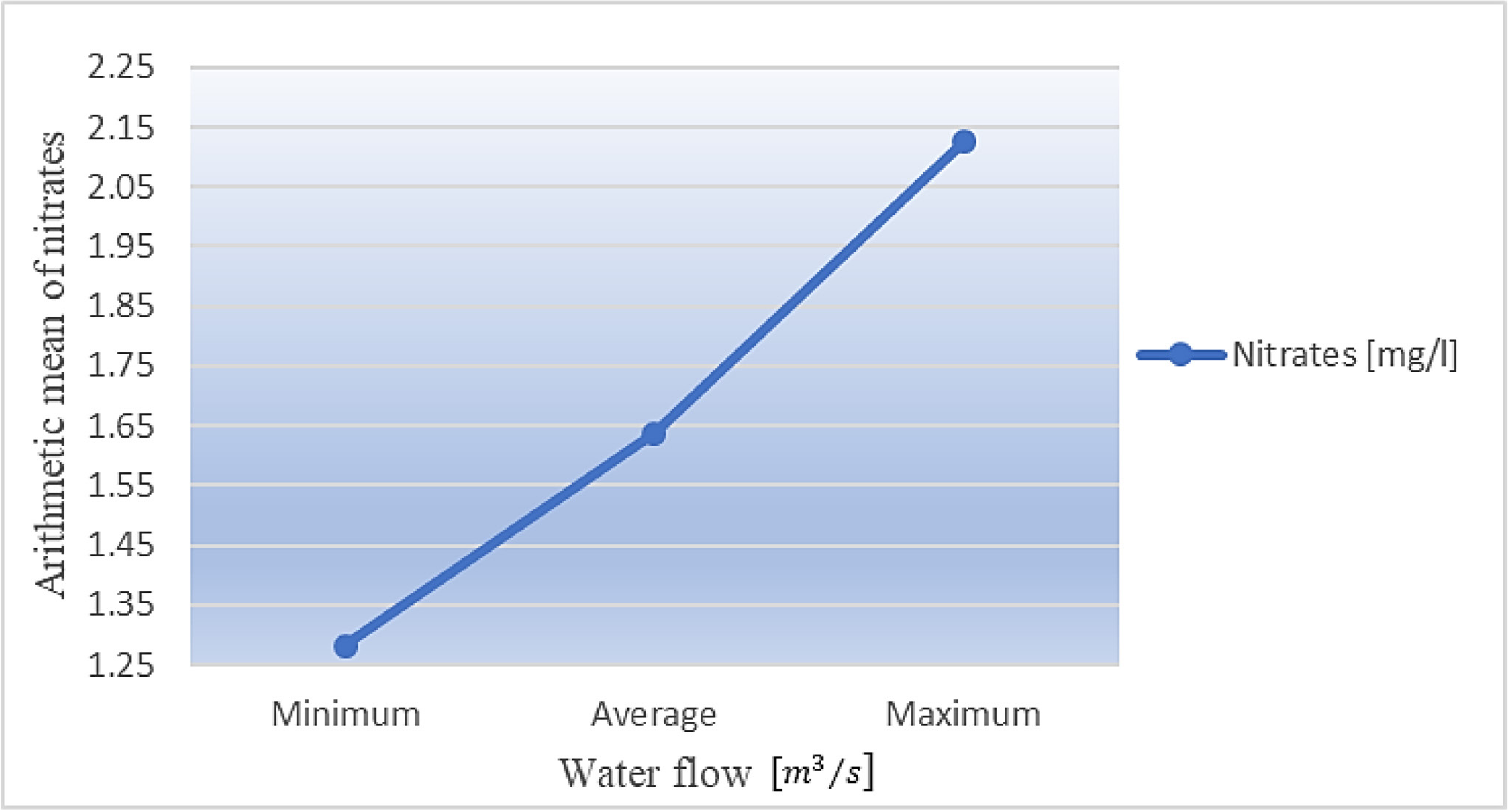
Furthermore, there is a difference in the concentration of nitrites in water depending on the intensity of water flow, F (2, 1606) = 50.85, p = 0.00. The concentration of nitrites in water during minimum water flow (M = 0.002 mg/l, SD = 0.002) is lower compared to the average intensity of water flow (M = 0.004 mg/l, SD = 0.004) and maximum water flow (M = 0.004 mg/l, SD = 0.003); p < 0.05. The difference in nitrite concentration in water during maximum flow is not significantly different from the concentration of nitrites during average flow; p > 0.05.
A difference in the concentration of iron in water was observed depending on the intensity of water flow, F (2, 1585) = 72.53, p = 0.00. The concentration of iron in water during maximum water flow (M = 0.35 mg/l, SD = 0.41) is higher compared to when there is minimum water flow (M = 0.12 mg/l, SD = 0.19) and when there is an average intensity of water flow (M = 0.18 mg/l, SD = 0.25); p < 0.05. The concentration of iron in water during minimum water flow (M = 0.12 mg/l, SD = 0.19) is lower compared to the average intensity of water flow (M = 0.18 mg/l, SD = 0.25); p < 0.05.
There is a difference in the concentration of manganese in water depending on the intensity of water flow, F (2, 1592) = 54.47, p = 0.00. The concentration of manganese in water during maximum water flow (M = 0.13 mg/l, SD = 0.21) is higher compared to when there is minimum water flow (M = 0.04 mg/l, SD = 0.07) and when there is an average intensity of water flow (M = 0.06 mg/l, SD = 0.11); p < 0.05. The concentration of manganese in water during minimum water flow (M = 0.04 mg/l, SD = 0.07) is lower compared to the average intensity of water flow (M = 0.06 mg/l, SD = 0.11); p < 0.05. A difference in water pH values was observed depending on the intensity of water flow, F (2, 1762) = 6.12, p = 0.00. The water pH value during minimum water flow (M = 7.84, SD = 0.24) is lower compared to when there is maximum water flow (M = 7.89, SD = 0.26) and when there is an average intensity of water flow (M = 7.89, SD = 0.23); p < 0.05. The water pH value during maximum water flow does not significantly differ from the average intensity of water flow; p > 0.05. There is a significant difference in water colour depending on the intensity of water flow, F (2, 1690) = 271.97, p = 0.00. Figure 6 presents fluctuations in water quality parameters concerning flow intensity, specifically focusing on colour.
The average water colour concentration
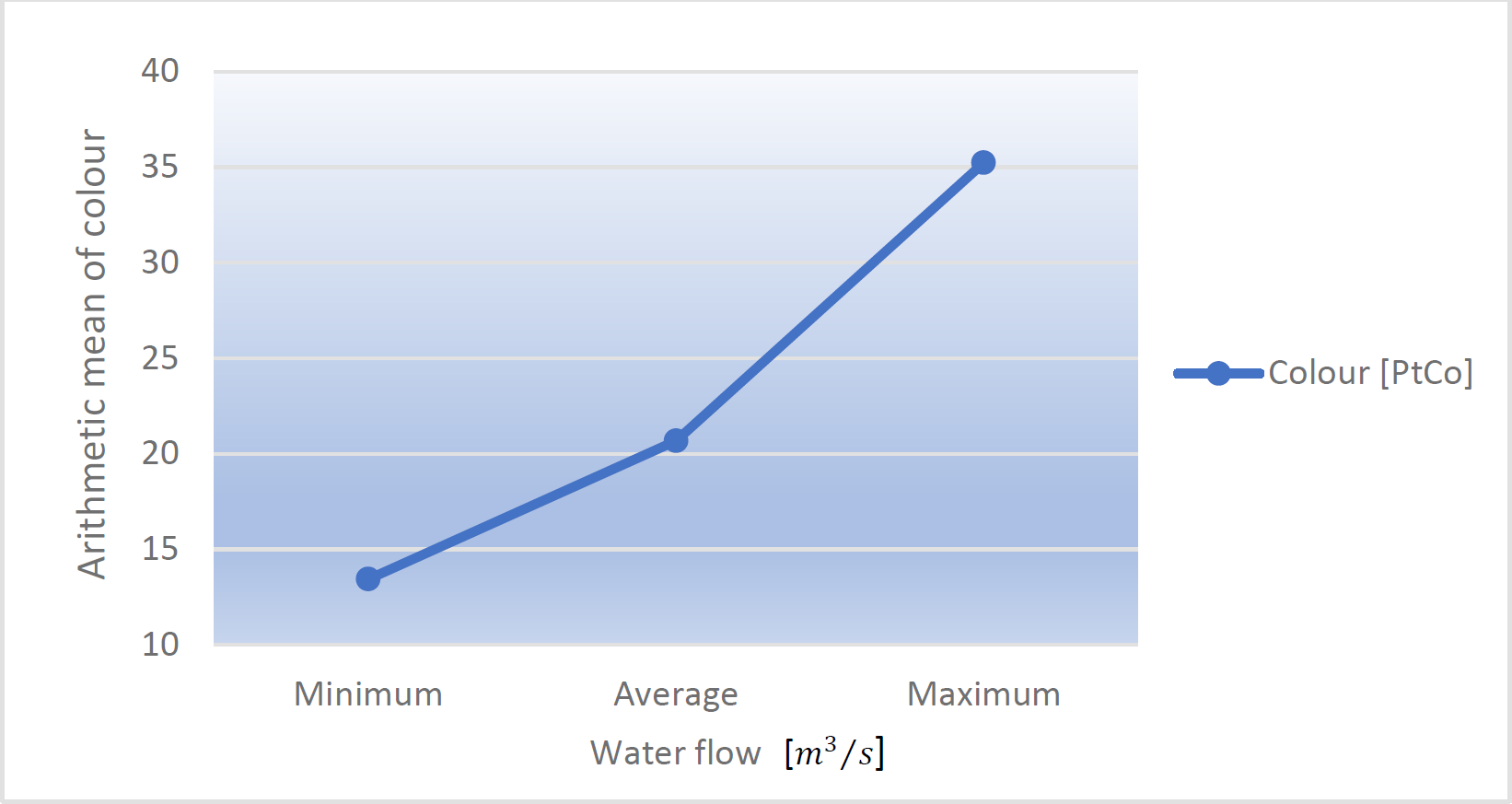
The water colour during maximum water flow (M = 35.23 PCU, SD = 17.54) is more pronounced than that when there is minimum water flow (M = 13.43 PCU, SD = 10.71) and when there is an average intensity of water flow (M = 20.69 PCU, SD = 12.93); p < 0.05. The water colour during minimum water flow is less pronounced than the average intensity of water flow; p < 0.05.
There is a difference in the concentration of aluminium in water depending on the water flow intensity, F (2, 1589) = 26.60, p = 0.00. The concentration of aluminium in water during maximum water flow (M = 0.19 mg/l, SD = 0.12) is higher compared to when there is an average water flow (M = 0.17 mg/l, SD = 0.09) and when there is minimum water flow (M = 0.15 mg/l, SD = 0.07); p < 0.05. The difference in aluminium concentration in water between average (M = 0.17 mg/l, SD = 0.09) and minimum water flow (M = 0.15 mg/l, SD = 0.07) is significant; p > 0.05.
The study's findings regarding the impact of extreme hydrological events on drinking water quality in rural areas, particularly in south-eastern Serbia, underscore significant insights into the relationship between water flow intensity and various water quality parameters. Specifically, the study delves into the variations observed in parameters such as turbidity, nitrates, COD, manganese, iron, and water colour concentration concerning fluctuations in water flow intensity within the Vlasotince region.
Contrary to conventional expectations, wherein heightened water flow is presumed to consistently elevate pollutant concentrations, the study reveals a nuanced understanding. It suggests that the relationship between water flow intensity and pollutant levels is not straightforward. While certain pollutants, notably turbidity and nitrates, exhibit higher concentrations with increased flow, others do not demonstrate a direct correlation.
Higher turbidity levels were observed during maximum water flow conditions. The increased turbidity can be attributed to the higher rate of runoff during heavy precipitation events, which tends to carry more suspended particles into the water system. High turbidity levels during the maximum water flow can impede disinfection processes, making it more challenging to eliminate pathogens effectively [46]. Therefore, monitoring and managing turbidity levels is essential to ensure water quality and protect public health. Management strategies to reduce turbidity could include erosion control measures, reforestation, and maintaining vegetation buffers around water bodies to trap sediments.
Similarly, significant variations were observed in nitrate concentration regarding water flow intensity. The nitrate concentration was higher during maximum water flow than at minimal and average flow intensities. Excessive nitrate levels in water bodies can lead to eutrophication, disrupting the ecological balance and promoting the growth of harmful algal blooms. Elevated nitrate concentrations during high-flow conditions could result from surface runoff containing agricultural fertilisers. Strategies to reduce nitrate pollution could involve implementing better agricultural practices, such as optimised fertiliser application schedules and establishing riparian zones [47]. Additionally, nitrate concentration during minimal flow was lower than the concentration corresponding to the average flow intensity.
The concentration of iron in water exhibited a significant difference depending on the intensity of water flow. Specifically, the concentration of iron was higher during maximum flow compared to both minimal and average flow intensities. Furthermore, the concentration of iron during minimal flow was lower than at the average flow intensity. Although iron is generally considered beneficial and less toxic than other heavy metals, it is crucial to acknowledge that its toxicity is dose-dependent. Iron can induce oxidative stress at elevated concentrations, leading to cellular damage and impairments in vital biological functions [48]. A significant difference was observed in manganese concentration depending on water flow intensity. Higher concentrations of manganese in water can cause a noticeable colour, odour, or taste in water. The increased iron and manganese levels during high flows could be due to the disturbance of sediments where these elements are present. To mitigate this, controlling soil erosion and avoiding activities that disturb the riverbed during high flows might be effective [49]. The study found negligible changes in pH value based on the intensity of water flow, suggesting that pH may be influenced by other factors not directly related to flow intensity. It is essential to note that the specific causal relationships between water flow and pollution concentrations can be complex and influenced by various factors, including land use, vegetation cover, soil type, and human activities. The study recommends long-term monitoring and extensive evaluations to better understand these relationships and develop targeted mitigation strategies for preserving optimal water quality.
Among other stressors, climate change exacerbates existing water challenges and introduces new uncertainties, including altered precipitation patterns, more frequent extreme weather-related events, and consequent intensive river volumetric flow oscillations. Adapting public water supply systems to future impacts necessitates proactive measures such as adaptation to extreme hydrological events influencing raw water quality parameters to enhance resilience and ensure reliable water access in a changing climate. In that sense, addressing the variability of raw water resources for public water supply from a global perspective underscores the need for collaborative efforts and innovative solutions, in which the predictive system described in this paper, coupled with smart measuring-information systems, can be seen as a contribution to the technical and organisational improvement of pre-water treatment operations [50]. In summary, while increased water flow naturally has the potential to raise pollutant concentrations due to greater runoff and erosion, strategic management and mitigation efforts can counteract this effect. These efforts include land use planning, agricultural practices, reforestation, and maintaining natural vegetation, which not only help manage water quality but also contribute to the overall resilience of the watershed against pollution.
According to the latest IPCC report, climate change is expected to exacerbate the frequency and severity of extreme weather events, such as heavy precipitation, floods, and droughts. These changes are likely to impact water quality by increasing pollutant loads, sediment transport, and the occurrence of algal blooms. The alteration in water temperature and flow regimes may also affect the biogeochemical processes in water bodies, leading to water management and treatment challenges. Extreme hydrological events, combined with seasonal variations, as well as changes in water use during the pandemic, such as the coronavirus pandemic and the already persistently high levels of poverty and inequity, represent a threat to the progress and water demands of the world's rural population. However, the pandemic has also demonstrated that new technologies can enable rural people to prosper, thereby crossing the rural-urban divide, which is fundamental to achieving the SDGs. Hence, enhancing the availability of clean drinking water promotes personal welfare and propels societal advancement in attaining objectives for environmental sustainability. In light of the emerging challenges posed by climate change, Serbia is poised to implement a range of strategic measures to adapt to its evolving climate conditions and minimise potential adverse effects. These multifaceted adaptation measures encompass various sectors, including water sectors, to address the diverse impacts of climate change. These measures include enhancement of water storage capacity and distribution systems to effectively manage the anticipated shifts in precipitation patterns, including increased rainfall intensity and prolonged drought periods, as well as improvement of irrigation practices and the utilisation of advanced technologies to optimise water use in agriculture.
To summarise, the outcomes of this research demonstrate that the water flow intensity impacts diverse water parameters. These findings underscore the crucial role of flow dynamics in water quality assessment, particularly during extreme hydrological events.
This study lays the groundwork for developing advanced tools to improve water resource management. By identifying these crucial relationships between flow and water quality, early warning systems can be built that predict water quality issues based on real-time or forecasted hydrological data. Additionally, decision support models can be developed utilising this information to help water managers explore and evaluate different response strategies, such as treatment adjustments or alternative water source utilisation, during critical events.
It is important to emphasise that the study's conclusions are limited to the analysed area (Vlasotince, Serbia) and the five-year study period (2014−2018). More research is necessary to validate these findings in other geographical locations and under diverse environmental conditions. Long-term monitoring and comprehensive evaluations are recommended to assess the long-term effects of flow intensity on water quality parameters and establish targeted mitigation strategies for preserving optimal water quality.
This study contributes to a deeper understanding of the complex interplay between extreme hydrological events and water quality parameters. It lays the groundwork for future research and informs sustainable water management practices. Identifying crucial relationships between flow and water quality paves the way for developing advanced tools, such as early warning systems and decision support models, to improve water resource management and support informed decision-making during critical events.
It is gratefully acknowledged that part of this research was financially supported by the Republic of Serbian Ministry of Science, Technological Development and Innovation. The authors would also like to express their appreciation for the efforts of the journal Editor and anonymous reviewers whose comments and suggestions have helped to improve the quality of the presented subject matter.
Abbreviations | |
---|---|
ANOVA | Analysis of Variance |
COD | Chemical Oxygen Demand |
EU | European Union |
HSD | Honestly Significant Difference |
NTU | Nephelometric Turbidity Unit |
pH | Potential of Hydrogen |
PCU | Platinum Colour Unit |
SD | Standard Deviation |
SDG | Sustainable Development Goal |
Changes in water use and wastewater generation influenced by the COVID-19 pandemic: A case study of China ,J Environ Manage , Vol. 314 ,pp 115024 , 2022, https://doi.org/https://doi.org/10.1016/j.jenvman.2022.115024
, - World Social Report 2021: Reconsidering Rural Development, 2021, http://desapublications.un.org/publications/world-social-report-2021-reconsidering-rural-development, Accessed: Nov. 29, 2023. [Online]. Available:
- Rural Europe, 2022, https://ec.europa.eu/eurostat/statistics-explained/index.php?title=Rural_Europe&stable=1, [Online]. Available:
- World Bank Open Data - Rural Population, https://data.worldbank.org, [Accessed: Mar. 25, 2023]
Environmental impact of water supply and water use in a Mediterranean water stressed region ,Journal of Cleaner Production , Vol. 88 ,pp 196–204 , 2015, https://doi.org/https://doi.org/10.1016/j.jclepro.2014.04.076
, An Environmental Performance Analysis of Upgraded and Integrated Treatment Techniques for Drinking Water Production through Reclamation ,[Journal of Sustainable Development of Energy, Water and Environment Systems] , Vol. [10] ([2]),pp [1]-[24] , 2022, https://doi.org/https://doi.org/10.13044/j.sdewes.d9.0393
, Research and innovation missions to transform future water systems ,Nat Water , Vol. 1 (3),pp Art. no. 3 , 2023, https://doi.org/https://doi.org/10.1038/s44221-023-00049-w
, - , Climate Change 2022 – Impacts, Adaptation and Vulnerability: Working Group II Contribution to the Sixth Assessment Report of the Intergovernmental Panel on Climate Change, 1st ed., 2023
Hydrological Regime Alteration Assessment in the Context of WFD 2000/60: A European and Global Review ,Sustainability , Vol. 15 (22),pp Art. no. 22 , 2023, https://doi.org/https://doi.org/10.3390/su152215704
, The Effect of Rainfall on Aquatic Nitrogen and Phosphorus in a Semi-Humid Area Catchment, Northern China ,International Journal of Environmental Research and Public Health , Vol. 19 (17),pp Art. no. 17 , 2022, https://doi.org/https://doi.org/10.3390/ijerph191710962
, Influences of key factors on river water quality in urban and rural areas: A review ,Case Studies in Chemical and Environmental Engineering , Vol. 8 ,pp 100424 , 2023, https://doi.org/https://doi.org/10.1016/j.cscee.2023.100424
, Simulation of the Potential Impacts of Projected Climate and Land Use Change on Runoff under CMIP6 Scenarios ,Water , Vol. 15 (19),pp Art. no. 19 , 2023, https://doi.org/https://doi.org/10.3390/w15193421
, Increasingly frequent extreme weather events urge the development of point-of-use water treatment systems ,npj Clean Water , Vol. 5 (1),pp Art. no. 1 , 2022, https://doi.org/https://doi.org/10.1038/s41545-022-00182-1
, Future changes in snowpack will impact seasonal runoff and low flows in Czechia ,Journal of Hydrology: Regional Studies , Vol. 37 ,pp 100899 , 2021, https://doi.org/https://doi.org/10.1016/j.ejrh.2021.100899
, Effects of change in streamflow patterns on water quality ,Journal of Environmental Management , Vol. 302 ,pp 113991 , 2022, https://doi.org/https://doi.org/10.1016/j.jenvman.2021.113991
, Impacts of extreme hydrological events on sustainable water resources management and human well-being ,Safety Engineering , Vol. 9 (1), 2019, https://doi.org/https://doi.org/10.7562/SE2019.9.01.06
, Screening and mitigating major threats of regional development to water ecosystems using ecosystem services as endpoints ,Journal of Environmental Management , Vol. 293 ,pp 112787 , 2021, https://doi.org/https://doi.org/10.1016/j.jenvman.2021.112787
, Rural–urban water struggles: urbanising hydrosocial territories and evolving connections, discourses and identities ,Water International , Vol. 44 (2),pp 81–94 , 2019, https://doi.org/https://doi.org/10.1080/02508060.2019.1583311
, Holistic water quality dynamics in rural artificial shallow water bodies ,Journal of Environmental Management , Vol. 223 ,pp 676–684 , 2018, https://doi.org/https://doi.org/10.1016/j.jenvman.2018.06.076
, Understanding the impact of the changes in weather conditions on surface water quality ,Science of The Total Environment , Vol. 652 ,pp 289–299 , 2019, https://doi.org/https://doi.org/10.1016/j.scitotenv.2018.10.246
, Quantifying climate, streamflow, and watershed control on water quality across South-eastern US watersheds ,Science of The Total Environment , Vol. 739 ,pp 139945 , 2020, https://doi.org/https://doi.org/10.1016/j.scitotenv.2020.139945
, Extreme weather events: Should drinking water quality management systems adapt to changing risk profiles? ,Water Research , Vol. 85 ,pp 124–136 , 2015, https://doi.org/https://doi.org/10.1016/j.watres.2015.08.018
, Extreme Hydrometeorological Events Influence to Water Quality of Small Rivers in Urban Area: A Case Study in Northeast Poland ,Sci Rep , Vol. 10 (1),pp Art. no. 1 , 2020, https://doi.org/https://doi.org/10.1038/s41598-020-67190-4
, Managing impacts of extreme hydrological events on urban water services: the Australian experience ,International Journal of Water Resources Development , Vol. 37 (6),pp 907–928 , 2021, https://doi.org/https://doi.org/10.1080/07900627.2020.1808447
, Cross-Scaling Approach for Water-Flow-Regulating Ecosystem Services: A Trial in Bochum, Germany ,Land , Vol. 11 (5),pp Art. no. 5 , 2022, https://doi.org/https://doi.org/10.3390/land11050740
, During a winter of storms in a small UK catchment, hydrology and water quality responses follow a clear rural-urban gradient ,Journal of Hydrology , Vol. 545 ,pp 463–477 , 2017, https://doi.org/https://doi.org/10.1016/j.jhydrol.2016.12.037
, Assessment of hydrological response to multi-year drought: Insights from lag characteristics and shift magnitude ,Hydrological Processes , Vol. 36 (7),pp e14636 , 2022, https://doi.org/https://doi.org/10.1002/hyp.14636
, Strategies for identifying pollution sources in a headwater catchment based on multi-scale water quality monitoring ,Environ Monit Assess , Vol. 193 (4),pp 169 , 2021, https://doi.org/https://doi.org/10.1007/s10661-021-08930-5
, Individual and combined impacts of future land-use and climate conditions on extreme hydrological events in a representative basin of the Yangtze River Delta, China ,Atmospheric Research , Vol. 236 ,pp 104805 , 2020, https://doi.org/https://doi.org/10.1016/j.atmosres.2019.104805
, Regional Pattern of Quality Characteristics of Groundwater in Oyo State, Nigeria Using ANOVA and Duncan's Post Hoc Test ,Journal of Hydrogeology & Hydrologic Engineering , Vol. 8 (2),pp 1–12 , 2019
, Statistical Analysis of Water Quality Parameters of Veliki Bački Canal (Vojvodina, Serbia) in the Period 2000-2009 ,Carpathian Journal of Earth and Environmental Sciences , Vol. 7 ,pp 255–264 ,
, An assessment of water quality in the Coruh Basin (Turkey) using multivariate statistical techniques ,Environ Monit Assess , Vol. 187 (11),pp 721 , 2015, https://doi.org/https://doi.org/10.1007/s10661-015-4904-9
, Assessment of seasonal and polluting effects on the quality of river water by exploratory data analysis ,Water Research , Vol. 32 (12),pp 3581–3592 , 1998, https://doi.org/https://doi.org/10.1016/S0043-1354(98)00138-9
, Application of Multivariate Statistical Methods to Optimise Water Quality Monitoring Network with Emphasis on the Pollution Caused by Fish Farms ,Iran J Public Health , Vol. 46 (1),pp 83–92 , 2017
, Influence of Land Use Land Cover on River Water Quality in Rural North Wales, UK ,JAWRA Journal of the American Water Resources Association , Vol. 57 (3),pp 357–373 , 2021, https://doi.org/https://doi.org/10.1111/1752-1688.12904
, - Delineation of surface water bodies dashboards, https://water.europa.eu/freshwater/resources/water-framework-directive-surface-water-data-products/delineation-surface-water, [Accessed: Apr. 14, 2024]
A new broad typology for rivers and lakes in Europe: Development and application for large-scale environmental assessments ,Science of The Total Environment , Vol. 697 ,pp 134043 , 2019, https://doi.org/https://doi.org/10.1016/j.scitotenv.2019.134043
, An Integrated Approach in the Assessment of the Vlasina River System Pollution by Toxic Elements ,Frontiers in Environmental Science , Vol. 10 , 2022, https://doi.org/https://doi.org/10.3389/fenvs.2022.909858
, - File:Serbia in Europe (-rivers -mini map).svg - Wikipedia, https://commons.wikimedia.org/wiki/File:Serbia_in_Europe_(-rivers_-mini_map).svg, [Accessed: Nov. 29, 2023]
- RHMS - Republic Hydrometeorological Institute of Serbia, https://www.hidmet.gov.rs/ciril/hidrologija/index.php, [Accessed: Mar. 25, 2023]
Trophic relations between macroinvertebrates in the Vlasina river (Serbia) ,Archives of Biological Sciences , Vol. 58 (2),pp 105–114 , 2006, https://doi.org/https://doi.org/10.2298/ABS0602105P
, - Final results of the Census of Population, Households and Dwellings, 2022 | Statistical Office of the Republic of Serbia, https://www.stat.gov.rs/en-US/vesti/20230428-konacnirezpopisa, [Accessed: Nov. 29, 2023]
- Tourism Development Plan of the Municipality of Vlasotince, 2011, https://vlasotince.rs/wp-content/uploads/2018/11/Vlasotince-plan_razvoja_turizmaA4-06.09.2011.pdf, [Accessed: Apr. 14, 2024]
- , "Nerezine" Vlasotince, Elaborate on the zones of sanitary protection of the water supply source in Vlasotince, 2012
Spatial Variations of the Water Quality Parameters in Basra Water Treatment Plants Using ANOVA ,Design Engineering (Toronto) , Vol. 2021 ,pp 3684–3703 , 2021
, - Water quality and health: Review of turbidity, https://www.who.int/publications-detail-redirect/WHO-FWC-WSH-17.01, [Accessed: May 25, 2023]
- Nitrate and Nitrite in Drinking-water, 2016, https://cdn.who.int/media/docs/default-source/wash-documents/wash-chemicals/nitrate-nitrite-background-jan17.pdf?sfvrsn=1c1e1502_4, [Accessed: Apr. 14, 2024]
- Determination of Iron Content in Water, 2017, https://opus.govst.edu/capstones/348, [Accessed: Apr. 14, 2024]
- Manganese in Drinking Water - MN Dept. of Health, 2021, https://www.health.state.mn.us/communities/environment/water/contaminants/manganese.html, [Accessed: Nov. 29, 2023]
Smart Water Technology for Efficient Water Resource Management: A Review ,Energies , Vol. 13 (23),pp Art. no. 23 , 2020, https://doi.org/https://doi.org/10.3390/en13236268
,